Sie befinden sich hier
Inhalt
Introduction
We established an operant conditioning paradigm with subjects learning to differentially regulate the BOLD effects in the right anterior cingulate cortex (rACC) and the left posterior Insula (pInsL). The aim was to investigate whether it is possible to regulate activation in these pain processing areas in response to painful stimulation in a way that uncouples the response in these functionally related areas.
Methods
We measured 20 healthy subjects, split into two groups 10 subjects each.
Group 1:
mean age of 29 years (SD = 6.48, range: 20–41), four females (M = 27.0, SD = 3.92), and six males (M = 30.3, SD = 7.81)
Group 2:
mean age of 28 years (SD = 4.71, range: 22 - 35), six females (M = 29.0, SD = 5.25) and four males (M = 26.0, SD = 3.65)
In the baseline session we applied painful electrical stimuli (approximately 70% above pain threshold) to the first segment of the fourth digit of the right hand using a stainless steel concentric bipolar needle electrode resulting in a clearly online localizable BOLD change in both the rACC and the pInsL (see Figure 1).
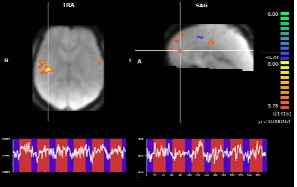
On four consecutive days seven days after the baseline session, subjects completed six feedback sessions, each lasting 7.21 minutes for the four conditions (24 sessions total, six sessions per condition). Visual feedback was given in the form of a blue or yellow ball, its movement indicating magnitude and direction of difference in BOLD activation in the rACC and pInsL parallel to the painful stimulation. Visual feedback of activation data was done with Presentation according to [1]

In each session subjects were first instructed to move the ball up or down during six painful stimulation blocks lasting 30 s in the direction of an arrow that appeared next to the ball during stimulation. In the 20 s between each stimulation block subjects performed mental arithmetic.
Rt-fMRI Data Acquisition
The fMRI data were acquired on a SIEMENS 3 T MAGNETOM Trio whole body MR scanner using a standard 12-channel head coil. Shimming of the scanner was done manually to account for maximum magnetic field homogeneity. A standard gradient field map was recorded at the beginning of each measurement. For acquisition of fMRI data, an EPI sequence (TR = 1500 ms, TE = 22 ms, matrix size = 96 x 96, flip angle = 90°, BW = 1270 Hz/px, parallel acquisition technique GRAPPA acceleration factor 2) was used. The EPI sequence was modified by inserting a real-time data export functor into the EPI post processing pipeline immediately before the MosaicDecorator functor. The complete volume was converted into ANALYZE format accessible to a separate computer connected by LAN. Twenty-four AC/PC aligned slices were acquired (voxel size = 2.2 x 2.2 x 3.5 mm3, gap = 0.5 mm). To avoid partial volume effects, a high in-plane resolution was chosen. To compensate for the reduction in signal-to-noise ratio resulting from this a nonisotropic voxel size was necessary. A 3D fast low angle shot high-resolution T1-weighted anatomical scan was recorded from each subject (TR = 23 ms, TE = 5.02 ms, matrix size = 448 x 448, flip angle = 25°, BW = 190 Hz/px, voxel size = 0.5 x 0.5 x 1.0 mm3) as anatomical reference. To reduce movement of the head, foam pegs were used to immobilize the subject’s head during the scanning periods. All steps of the training, scanning, and feedback procedures were always performed by the same person to insure maximum comparability between all scans.
Online Analysis of the fMRI Data
Online data analysis was performed with Turbo Brain-Voyager Version 1.1 as described in [2]. Online preprocessing of the functional data included incremental linear detrending, 3D motion detection and correction and drift removal. A recursive least squares regression algorithm was used for correlation analyses, for the linear detrending a linear predictor was added as a confound to the GLM. The feedback signal reflects the unrelated region corrected percent signal change of the BOLD signal in the ROI. The software computes statistical maps from scan to scan based on the GLM. Feedback computation and visualization was performed with in-house written scripts based on Presentation on another computer connected with Turbo BrainVoyager via LAN.
Offline Analyses of the fMRI Data
Offline data preprocessing of the fMRI scans was performed using BrainVoyager QX 2.3. Gradient field map correction using the BrainVoyager plugin was performed on the EPI images to reduce geometric distortions and improve spatial accuracy to maximize fMRI sensitivity [3]. Offline preprocessing comprised 3D motion correction (linear interpolation for motion detection and windowed sinc interpolation for motion correction). The datasets were then spatially smoothed with a Gaussian kernel with a full width half maximum of 8 mm3. Linear detrending was performed before high frequency artifacts were removed applying a highpass filter (0.006 Hz, approximately 2.5 times the duration of a combined regulation and nonregulation phase). A response function was estimated by convoluting a condition box-car timecourse with a standard hemodynamic response function (two-gamma HRF).
All functional datasets were coregistered with their respective anatomical dataset. The anatomical datasets were transformed into standard Talairach space using BrainVoyager. The anatomical transformation functions were then applied to the EPI datasets. All coregistration and transformation procedures were conducted manually to insure maximum fit and overlap of the datasets. A group analysis based on the increase in BOLD percent signal change during the regulation phase with respect to the nonregulation phase was performed using a GLM. All results were Bonferroni corrected for multiple testing. Offline time course data analysis of the feedback was automated with MATLAB.
References
[1] Weiskopf et al., IEEE Trans Biomed Eng, 2004, 51, pp. 966 - 970.
[2] Weiskopf et al., NeuroImage, 2003, 19, pp. 577 - 586.
[3] Fellner et al., Clin Hemorheol Microcirc, 2009, 43, pp. 71 - 82.
Further reading
- M. Bucolo, M. Rance, F. Nees, M. Ruttorf, G. Stella, N. Monarca, J. Andoh and H. Flor. Cortical networks underlying successful control of nociceptive processing using real-time fMRI. Front Pain Res, 2022, 3, 969867.
- M. Rance, M. Ruttorf, F. Nees, L.R. Schad and H. Flor. Neurofeedback of the difference in activation of the anterior cingulate cortex and posterior insular cortex: two functionally connected areas in the processing of pain. Front Behav Neurosci, 2014, 8, Article 357.
- M. Rance, M. Ruttorf, F. Nees, L.R. Schad and H. Flor. Real Time fMRI Feedback of the Anterior Cingulate and Posterior Insular Cortex in the Processing of Pain. Hum Brain Mapp, 2014, 35, 5784 - 5798.
- M. Bucolo, M. Rance, A. Muscarello, A. Spampinato, M. Ruttorf and H. Flor. Brain Connectivity Networks and fMRI Brain-Computer Interface. International Journal of Bioelectromagnetism, 2012, 14, 154 - 161.