Sie befinden sich hier
Inhalt
Innate Immunity in Critical Care
In critically ill patients, both the innate immune response to infection and to non-infectious tissue damage, e.g., due to surgery, can rapidly escalate to organ dysfunction and sepsis. This complicates sepsis biomarker discovery and makes reliable sepsis diagnosis in critical care a significant clinical challenge: While swift antimicrobial treatment is life saving for infected patients, unnecessary antimicrobial therapy fosters antimicrobial resistance in non-infected patients.
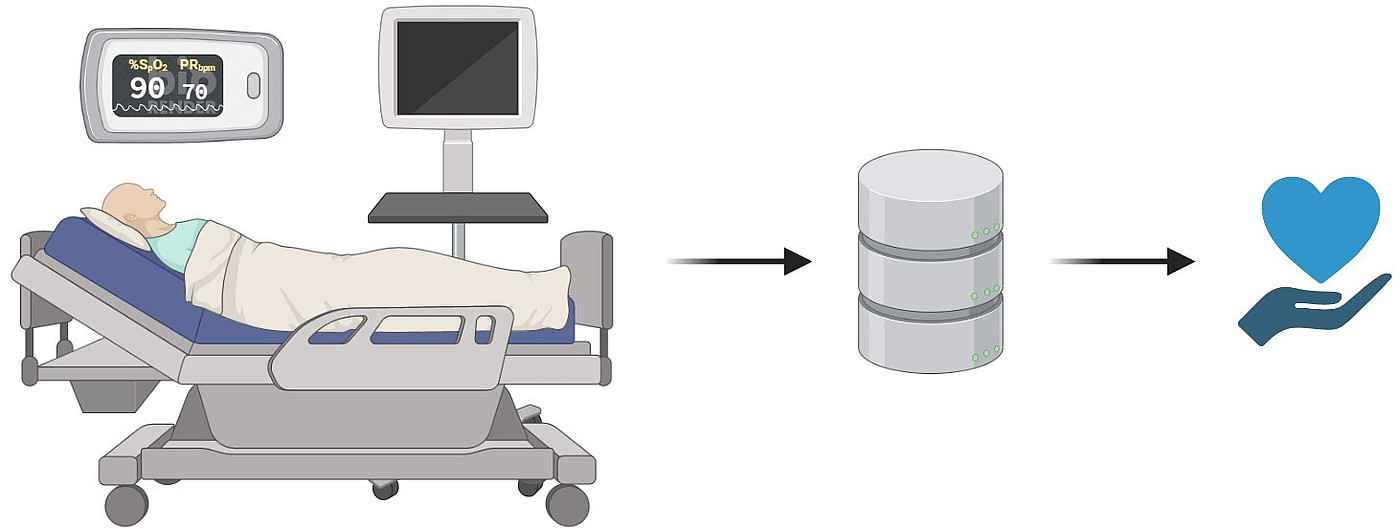
The clinical epidemiology group aims at more accurate sepsis prediction and diagnosis. We study the dynamics of the systemic inflammatory response syndrome (SIRS) in the electronic medical records of critically ill patients. Linking in-hospital data sources, we uncover treatment pathways of sepsis patients and, jointly with the experimental lab, prospectively explore the role of surgery as immunological stimulus (in the clinical cooperation unit digital data spaces in sepsis). We have developed an automated SIRS-algorithm and harness clinical expertise for valid determination of sepsis onset (ground truth). In addition to applying state-of-the-art epidemiology and biostatistics methods, we contribute to machine learning efforts.
Publications
Schefzik R, Boland L, Hahn B, Kirschning T, Lindner HA, Thiel M, Schneider-Lindner V
Differential network testing reveals diverging dynamics of organ system interactions for survivors and non-survivors in intensive care medicine.
Frontiers in Physiology 2022; 12:801622. doi: 10.3389/fphys.2021.801622
Lindner HA, Schamoni S, Kirschning T, Worm C, Hahn B, Centner F-S, Schoettler JJ, Hagmann M, Krebs J, Mangold D, Nitsch S, Riezler S, Thiel M, Schneider-Lindner V
Ground truth labels challenge the validity of sepsis consensus definitions in critical illness.
Journal of Translational Medicine 2022; 20:27. doi: 10.1186/s12967-022-03228-7
Schamoni S, Lindner HA, Schneider-Lindner V, Thiel M, Riezler S.
Leveraging implicit expert knowledge for non-circular machine learning in sepsis prediction.
Artif Intell Med. 2019;100:101725.
Lindner HA, Balaban Ü, Sturm T, Weiß C, Thiel M, Schneider-Lindner V.
An algorithm for systemic inflammatory response syndrome (SIRS) criteria-based prediction of sepsis in a polytrauma cohort.
Critical Care Medicine 2016; 44(12):2199-2207
Beier D, Weiß C, Hagmann M, Balaban Ü, Thiel M, Schneider-Lindner V.
Is antibacterial treatment intensity lower in elderly patients? A retrospective cohort study in a German surgical intensive care unit
BMC Health Serv Res. 2019;19(1):367. doi: 10.1186/s12913-019-4204-0.
Kontextspalte
Contact
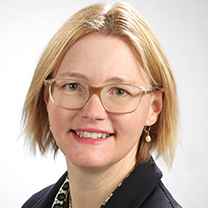
PD Dr. Dr. Verena Schneider-Lindner
Principle Investigator Clinical Epidemiology der Research Group TRACC,
Klinik für Anästhesiologie und Operative Intensivmedizin
Phone +49 621 383-2755
verena.schneider-lindner@ medma.uni-heidelberg.de