Sie befinden sich hier
Inhalt
Ultrasound Computed Tomography (USCT) is a novel imaging method with high potential for early breast cancer diagnosis. It promises high sensitivity and specificity for early breast cancer detection without the associated risks of ionizing radiation and lower costs than magnetic resonance tomography. The aim of the project is to investigate a new method for early breast cancer diagnosis: high-resolution transmission imaging via USCT. We consider various machine learning methods including deep learning strategies to help improve the imaging performance.
The image acquisition of USCT is based on ultrasound transducers surrounding the breast within a water bath (Figure 1 shows a typical USCT machine for clinical application). We investigate deep learning techniques to reconstruct high-resolution images according to the acquired data. The parts of reconstructed images are shown in Figures. 2-3.
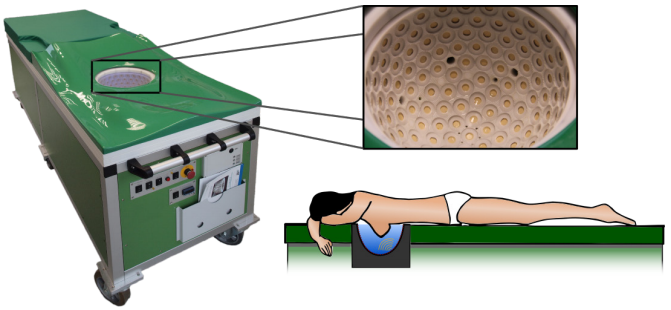
Project-related publications
[1] Y. Fan, H. Wang, H. Gemmeke, T. Hopp, J. Hesser. "DDN: dual domain network architecture for non-linear ultrasound transmission tomography reconstruction." Medical Imaging 2021: Ultrasonic Imaging and Tomography. Vol. 11602. International Society for Optics and Photonics, 2021.
[2] Y. Fan, H. Wang, H. Gemmeke, J. Hesser. "MI-Net: A Deep Network for Non-linear Ultrasound Computed Tomography Reconstruction." 2020 IEEE International Ultrasonics Symposium (IUS). IEEE, 2020.
[3] Y. Fan, H. Wang, H. Gemmeke, T. Hopp, K.W.A. van Dongen, J. Hesser. "Memory-Efficient Neural Network For Non-Linear Ultrasound Computed Tomography Reconstruction." 2021 IEEE 18th International Symposium on Biomedical Imaging (ISBI). IEEE, 2021.
[4] Y. Fan, H. Wang, H. Gemmeke, J. Hesser. Model-data-driven Image Reconstruction with Neural Networks for Ultrasound Computed Tomography Breast Imaging. Neurocomputing, 2021.
[5] W. Zhao, H. Wang, H. Gemmeke, K.W.A. van Dongen, T. Hopp, and J. W. Hesser. Ultrasound transmission tomography image reconstruction with fully convolutional neural network. Physics in Medicine & Biology, 2020.
Staffs:
Juergen Hesser, Wenzhao Zhao, Yuling Fan, Hongjian Wang, Hartmut Gemmeke, Torsten Hopp.